-
IV
- - Independent variables
- - Influence (influences another)
- - Cause / Predictor
- - Can be manipulated
- - Its's value does not depend on another variable - The value that is manipulated - For example: - Does "GENDER" have an influnce on depression? "Gender"= IV
-
DV
- - Dependent variable
- - Outcome / Effect
- - The value depends of the cause (independent variable)
- - The variable that is measured
-
Nominal
- - Categorical
- - Mutually Exclusive
- - Not ranked in order
- Examples:
- MEAL PREFERENCE: Breakfast, Lunch, Dinner
- RELIGIOUS PREFERENCE: 1 = Buddhist, 2 = Muslim, 3 = Christian, 4 = Jewish, 5 = Other
- 1 = male, 2 = female.
-
Ordinal
- - Categorical
- - Mutually Exclusive
- - Ranked in order
- Examples:
- RANK: 1st place, 2nd place,. last place
- LEVEL OF AGREEMENT: No, Maybe, Yes
-
Interval
- - Continuous
- - Scale
- - No absolute zero
- - Scale responses- NO true meaning between scores
- Examples: TIME OF DAY on a 12-hour clock -
- What is your bed time? 8-9; 9-10; 10-11; 11-12
-
Ratio
- - Continuous
- - Scale
- - Absolute zero
- - Scale responses: "HAVE" true meaning between scores
- Examples: INCOME: money earned last year
- NUMBER of children
- GPA: grade point average
-
Example Question:
Level of measurement
- Are you serious about attending a post crisis workshop? Yes/No
- Do you believe in the death penalty? Yes/No
- What is your favorite color? Blue/ Green/Red
Nominal
-
Example Question: Level of measurement
- How serious are you about attending a post crisis workshop? Extremely serious/very serious/somewhat serious/ not serious
- How satisfied are you with the MSW program at CSULB? Very satisfied, Somewhat satisfied, Not satisfied
Ordinal
-
Example Question: Level of measurement
On a scale from 1-10, respond to how serious you are about attending a post crisis workshop? 1=not at all; 10=extremely serious
- The death penalty is justified in certain cases? 5:Strongly agree; 4:Agree; 3: Neutral; 2:Disagree; 1: Strongly disgree
Interval
-
Example Question: Level of measurement
- How many clients have you had in the last six months?
- How many days would you attend a 20 day post crisis workshop if offered?
Ratio
-
Measures of Central Tendency
- - Univariate Analyses
- - Descriptive Statistics (describes something about your populations)
- - Mode = Most frequent score
- - Median = Mid point (n+1/2), # line
- - Mean = Averge (add scores togther divide by x)
-
Measures of Dispersion/Variability
- - Univariate Analyses
- - Descriptive Statistics (describes something about your populations)
- - Range + Interquarrite Range (mid 50%) difference between the largest and smallest values (Take the largest score and subtracts from the smallest score)
- - Standard Diviation = Square root of s2 = +/- SD
- - Variance (s2)=
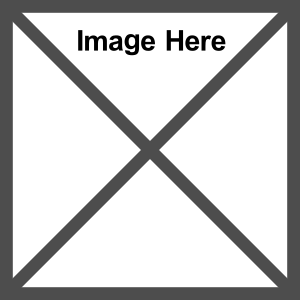
-
Null Hyothesis
- -Ho
- - If significance is found reject the Null (Ho)
- H o = pre means + post means
-
Alternative Hyothesis
- -Ha-
- If significance is found you fail to reject the Alternative (Ha) (accept Ha)
- H a = pre means ≠ means
-
Correlation (r) Relationships
- Ho --> r = 0
- Ha --> r ≠ 0
- -"p" value -
- -> Less than 0.05=Significance
- -> Greater than 0.05- NO Significance
- Phi, pb (Point Biserial), Cramer's V
-
P Value
- - Significance
- - How much possible evidence do we have aginst the null
"p" value:
- -> Less than 0.05 or equal to =Significance
- -> Greater than 0.05 or equal to- NO Significance
-
Cross Tabulation
- - Bivariate Analyses (2 variables)
- - Includes 2 catergorical variables
- Table to display cell count (2x2; 3x2)(define rows (R) and column (C))
-
Chi Square x 2
- - Bivariate Analyses (2 variables)
- - Includes 2 catergorical variables
- Test of ASSOCIATION (difference) between 2 catergorical variables
- Phi (Correlation) 2 + variables
-
Quantatative Research
- Univariate Analysis
- Bivariate Analysis
- Datad ananylses, results, findings, conclusions
- The use of surveys, scales, instruments, charts
-
t -test
- Bivariate Analyses
- - Catergorical IV (2 independent grps)
- - Categorical DV (mean scores (differnce))
- Test of ASSOCIATION (difference)
H o --> Mean score (boys) = Mean score (girls)
- Independent t -test-->
- - X (pre mean) ≠ X (post mean) (independent of each other)
- Dependent t -test--> PAIRED
- - X (pre mean) WITH X (post mean) (paired together)
- ANOVA--> one IV (3 means)
- - X boys (pre mean) ≠ X girls ≠ Xtrans
-
Relationships
(same as Independent t test except looking at realtionhsip)
- - pb - Point Biseral Correlation
- -->1 catergorical IV; 1 continuous DV
- -ETA (NOT ON TEST)
- -->2 catergoriacl IV's; 1 continuous DV
-
Hypothesis example
Chi Square X2:
- Ho : Odds = 1
- Ha : Odds ≠ 1
- ANOVA: Association
- Example: Depression vs girls vs boys vs transgender
H o: X boys = X girls = X transgender
H a: X boys ≠ X girls ≠ X transgender
- Independent t -test-->
- Ho: Xgirls = Xboys
- Ha: X girls≠ Xboys
- Dependent t -test-->
- Ho: Xpre mean = Xpost mean
- Ha: Xpre mean ≠ Xpost mean
|
|