-
-
Nominal Data
- values that correspond to unordered categories or classes
- eg. hair color, gender, state of birth, etc.
- have no ordering & are summarized as percentages
- nominal data that can take 1 of only 2 values (eg. having or not having gonorrhea are called binary/dichotomous data)
- type of Categorical data
-
Ordinal Data
- when the categories of a variable have an inherent order to them
- eg. Glasgow Coma Score, severity of adverse events (AE), “satisfaction”
- type of Categorical data
-
Discrete Data
- data restricted to specific integer values
- eg. number of children, bacteria count
- type of Measured data
-
Continuous Data
- unrestricted real numbers
- eg. weight, age, degree of stenosis, physician salary
- type of Measured data
-
Age as a Continuous & Ordinal Variable
- age itself can be reported using mean & standard deviation (SD)
- OR it can be grouped into categories - 45-54 yr, 55-64, ≥ 65 - & reported as percentage (x % were at this age, etc.)
-
Bar Charts
- useful for summarizing Categorical (nominal & ordinal) data
- displays # or % of individuals in each of the categories
- their bars do NOT touch to emphasize that the categories are DISTINCT from each other & MUTUALLY EXCLUSIVE
-
Histogram
- bars touch each other to emphasize the data are CONTINUOUS (measured)
- like in a bar chart, each bar of a histogram displays the # or % of individuals in each interval
- used to display the distribution of data in a sample
- is VERY good at displaying extreme data points
-
Histogram Example
y-axis = frequency (or %) - aka # of individuals whose cholesterol falls between 5 & 6
-
Arithmetic Mean (Average)
- sum of observations divided by the # of observations
- if one were to place “weight” on a numeric scale, the arithmetic mean would be at the balancing point
- because of this, it’s SENSITIVE to EXTREME observations (outliers)
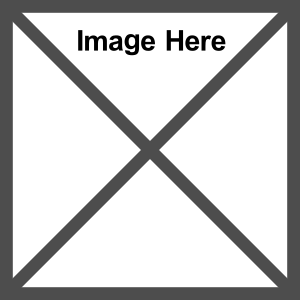
-
Median
- NOT sensitive to extreme observations
- the value that divides an ordered data set in half
- 50% of the data are ABOVE the median value, & 50% are below it
-
1st Quartile
refers to the value where 25% of the data are below it
-
3rd Quartile
the value where 75% of the data are below it
-
Interpret This Table (re: Quartiles)
- 25% of patients have an LVOT below 7 mmHg
- 50% of patients have an LVOT below 16 mmHg
- 75% of patients have an LVOT below 26 mmHg
- the interquartile range (7-26) contains 50% of the observations
-
Box Plot
- an illustrative tool for displaying the MEDIAN as well as the 1st & 3rd quartiles
- the Whiskers extend to the edge of extreme values in the data set
- more extreme values are individually displayed as dots
- can be useful when comparing 2 or more groups
-
Cholesterol & Grouped Box Plots
- even though women & men have similar distributions of cholesterol levels by age group, it’s easy to see that the median cholesterol levels increase as women age
- however levels remain relatively stable in men as they age
-
Distribution Shapes
when looking at distributions of data (using either histograms or box plots), it’s important to characterize them in regard to how far a distribution deviates from symmetry
-
Symmetrical Distribution
- when the mean, median, & mode have identical values
- normal distributions are symmetric
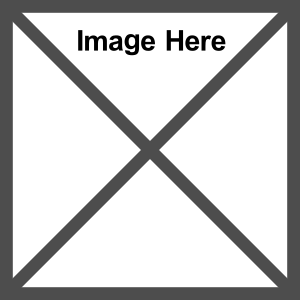
-
Positive Right Skew
- mean the skew is “to the right” - this manifests in a graph with a tail on the right side of the graph (is more “pronounced)
- mean > median
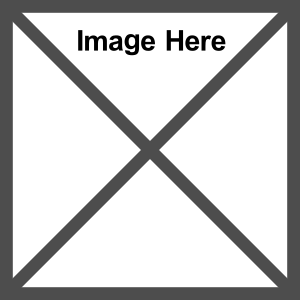
-
Negative Left Skew
- the skew is to the left
- the left “tail” is more pronounced
- mean < median
- Mode
- the most common (repeated) value in a dataset
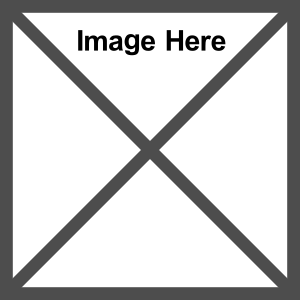
-
Skew with a Box Plot & Histogram
- Positive/Right Skew: both the right whisker & tail are ELONGATED
- Negative/Left Skew: both the left whisker & tail are ELONGATED
- Symmetrical: both whiskers & tails are equal distribution from the center
- Extremes: box plot displays them as individual data points; histogram displays them separately, with discontinuity
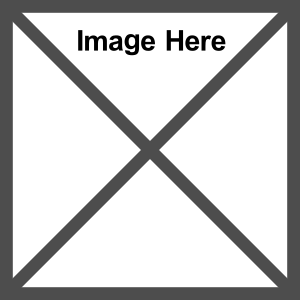
-
Standard Deviation
- numerically characterizes the amount of variability (data dispersion, data spread) among observed data points
- the mathematical formula calculates the average distance from the mean
- square root of the variance
-
Z Scores
- indicate how many SDs an observation is above or below the mean of a distribution
- computed by subtracting the mean from the observation & dividing the the SD
- Z = random variable - the mean / SD
- or (x - μ) / σ
-
What are the units of Z Score?
there are none because the mean & SD have the same units, the units cancel out
-
What are 3 important numbers to remember from a NORMAL distribution?
- 68
- 95
- 99
- ~ 68% of the observations lie within ±1 SD of the mean
- ~95% of samples lie within ±2 SDs of the mean
- ~99% lie within ±3 SDs of the mean
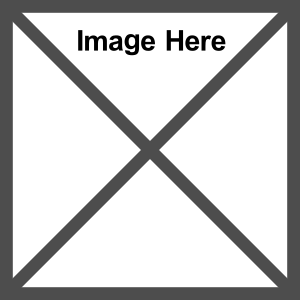 - someone with a Z score of 1 is 1 SD away from the mean
-
Chance
a probability expressed as a percentage
-
-
Target Population
all individuals we’re interested in studying
-
Random Sample
a representative subset chosen from said population of individuals we’re interested in studying
-
-
Standard Error
- SE = pop. standard deviation / √ sample size
- SE = σ / √n (eg. 41/√10)
-
Sampling Distribution of the Mean
describes the entire spectrum of sample means that could occur for all possible random samples of a given size n from means that are COMMON (near the center of the distribution/curve) to means that are RARE (near the edges of the distribution)
-
Central Limit Theorem
- given the existence of a population mean (μ) & population standard deviation (σ) from any distribution of any shape, the CLT states that the distribution of sample means (computed from random samples of size n from a population) will have its mean centered at the population mean
- the standard deviation of all the means = standard error
- if n > 30, the shape of the sampling distribution will be ~normal
- important because it forms the basis of all statistical tests
-
Confidence Interval
- used to approximate the population parameter of interest using a point estimate (eg. sample mean)
- a range of values that, with a known level of certainty, includes (surrounds, captures) the unknown population value
-
What does a 95% CI indicate?
that we are 95% confident that the range of values between the lower & upper limits contains the true population value
-
CI Formula
- point estimate ± critical value * SE(point estimate)
- critical value indicates a level of certainty (eg. 95%)
-
How to Interpret a CI
1. We are 95% confident that _______ levels (scores, values, etc.) ranging from x to y (units) capture the true mean _________.
2. We are 95% certain that the true mean ________ level is between x to y (units).
-
What 3 things can make a CI wider (aka less precise in capturing the true population value)?
1. Increasing the level of confidence (eg. 95% → 99%, you widen the CI to be more certain in capturing the true population value)
2. More variability among the observations (eg. larger standard error); more variability implies less precision in capturing the true pop. value
3. a smaller sample size (there’s less information involved in capturing the true population value)
-
What does the process of testing a hypothesis begin with?
specifying the null & alternative hypothesis
null: statistical statement that indicates no difference, response, or change exists
alternative: contradicts the null
-
α
- significance level of the test
- usually set at 5%
- represents the threshold beyond which the null hypothesis would be rejected in favor of the alternative hypothesis
-
p-value
- probability of obtaining a test-statistic at LEAST as extreme as the one that was actually observed, assuming that the null hypothesis is true
- can be interpreted as the likelihood that the observed result could’ve occurred by chance alone
-
T-test
- ratio of the observed mean difference to the amount of sampling variability (given via standard error)
- t values close to 0: support the null hypothesis
- t values further from 0: support the alternative hypothesis
- eg. T = 3.4 implies the mean difference is 3.4 standard errors ABOVE 0 (supports the alternative hypothesis)
-
How is the p-value calculated?
- it is the area under the sampling distribution of mean differences in both the L & R tails
- the areas that yield the p-value are calculated from a t distribution
- half the p-value comes from the L tail & half comes from the R tail
-
What does a p-value of 0.0007 mean?
it means that the alternative hypothesis is true & there’s a 0.07% chance that the difference could have occurred by chance alone
-
What kind of correspondence is there between p-values & confidence intervals?
1:1
whenever the CI doesn’t contain the parameter of interest, the null hypothesis can be rejected
eg. if a 95% CI about a mean difference EXCLUDES 0, we can conclude that the mean difference is significant at an alpha level of 5%
however, if a 95% CI about a mean difference INCLUDES 0, we cannot reject the null hypothesis
-
Chi Square Test
- used when the outcome & exposure variables are categorical
- eg. % of current smokers among newly diagnosed diabetes patients
- the basis of the chi-square test is to quantify the extent of agreement between the observed results gathered from data collection & the EXPECTED results one would observe if the null hypothesis were true
-
Interpretation of the Chi Square
- chi square values near 0: accept the null hypothesis
- large chi square values: reject the null hypothesis, accept the alternative hypothesis
- χ2 = 0.61 implies good agreement between observed & expected results → accept the null hyp.
- the p-value for a chi square test is calculated as a tail area under the chi square distribution
-
Type I Error (α)
- when the null hypothesis is rejected (alternative hypothesis accepted) when it shouldn’t have been
- a difference in the sample is observed when there is actually no difference in the population
- (guilty verdict when defendant is innocent)
-
Type II Error (β)
- when the null hypothesis is not rejected (alternative hypothesis is rejected) when it should have been rejected
- (not guilty verdict when defendant is guilty)
-
Power
- 1 - β
- the probability that a statistical test will RESULT in the REJECTION of the null hypothesis (acceptance of the alternative hypothesis) when it SHOULD be rejected
- (when a jury correctly assigns guilt)
-
When is power considered?
- 1. when a study is being planned, to determine the # of participants to enroll
- 2. when the null hypothesis is accepted (NOT rejected)
-
How can the power of a study be increased?
- 1. ↑ the expected effect size (eg. expected association, difference in means)
- 2. ↓ the expected standard error by:
- ↑ the sample size or ↓ standard deviation
-
What happens every time a statistical test is performed and a p-value is reported?
- a Type I error is made
- this is because the significance level for the test is pre- specified
- multiple testing inflates the overall Type I error rate
-
Overall Type I Error Rate
- 1 - (1 - α)^# of tests
- eg. if 5 tests are performed at α = .05 (5% sig level)
- error rate = 1–(1–0.05)^5 = 0.226 (22.6%)
- this means there’s a 22.6% chance of finding at least 1 significant difference when it doesn’t exist
- (instead of the usual 5% chance of a false-positive finding)
-
-
What are the 3 levels of screening?
- 1. Primary
- 2. Secondary
- 3. Tertiary
-
Primary Screening
- screening is done to prevent the disease
- eg. serum lipids are screened to prevent coronary artery disease
-
Secondary Screening
- the attempt to reduce the impact of the disease
- eg. mamography
-
Tertiary Screening
- aims to improve the quality of life of those with the disease
- eg. metabolic bone screen for pathologic fractures
-
What kinds of diseases are appropriate for screening?
those that are serious, common, & ones that would benefit from treatment before symptoms/sign’s develop
-
What’s important when selecting a screening test?
making sure it’s available, inexpensive, low risk, easily performed, reliable (it’s results can be reproduced), & accurate (results are correct)
-
Test Results
- people with a disease: true positives & false negatives
- people with no disease: true negatives & false positives
-
What are the two test performance measures?
- 1. Sensitivity
- 2. Specificity
- these are measures of test performance
-
Sensitivity
- true positives / true positives + false negatives
- calculated ONLY among individuals WITH the disease
- given the disease is present, the likelihood of testing positive
- TP / TP + FN
-
Specificity
- true negatives / true negatives + false positives
- calculated only among individuals WITHOUT the disease
- given the disease is not present, the likelihood of testing negative
- TN / TN + FP
-
Predictive Value Positive
- TP / TP + FP
- calculated ONLY among individuals that test positive
- the number of true positives divided by the sum of true & false positives
-
Predictive Value Negative
- TN / TN + FN
- calculated only among individuals that test NEGATIVE
- the number of true negatives divided by the sum of true & false negatives
-
Why does the “prior probability” (prevalence) of disease matter when interpreting test results?
because changes in prevalence can alter Predictive Value Positive & Predictive Value Negative
-
What happens to Predictive Value Positive & Predictive Value Negative as prevalence increases?
- predictive value positive increases
- predictive value negative decreases
-
What happens to Predictive Value Positive & Predictive Value Negative as prevalence decreases?
- predictive value positive decreases
- predictive value negative increases
-
What happens to Sensitivity & Specificity as prevalence changes?
they remain unaffected by prevalence!
-
Likelihood Ratio
a method that quantifies the likelihood that a given test result represents true disease or not
it’s the ratio of the chance that a certain test result will be found in a patient who has the disease versus the chance that the test result will be found in a patient who does not have the disease
-
Likelihood Ratio Formula
LR for a positive result = sensitivity / (1-specificity)
LR for a negative test result = (1-sensitivity) / specificity
- to calculate LR in general:
- # of people with a disease with a certain test outcome / # of people without a disease with the same test outcome
-
-
Randomized Control Trial (RCT)
- volunteers are randomized to:
- 1. experimental arm
- 2. placebo/standard/control arm
- both groups are followed over a certain period of time
- then the incidence of outcome in both groups is measured & compared
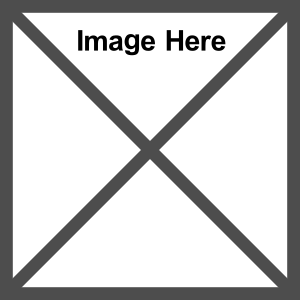
-
What are the 5 Steps of a RCT?
- 1. Enroll volunteers using strict inclusion/exclusion criteria
- 2. Allocate to treatment groups by randomization
- 3. Follow for relevant time period
- 4. Ascertain endpoint/outcome
- 5. Analyze results
-
Why are RCTs considered the “gold standard” of studies?
Randomization
-
Randomization
- reduces potential bias by:
- removing investigator treatment preference, volunteer treatment preference, & balances the trial arms
- it results in similar risk profiles in both groups
-
Blinding of Treatment
- investigators & volunteers don’t know whether a person received a treatment or not
- this prevents investigators & volunteers from biasing the results
- treatments should look alike (can be difficult if it’s say radiation v. chemotherapy) however the outcome/end points committee can be blind when they evaluate both groups
-
How does using a placebo reduce potential bias?
- it removes the “placebo effect” from the measure of treatment effect
- it removes volunteer influence by blinding
- it removes investigator influence by blinding
- these are especially important if the outcome is SUBJECTIVE
-
How long are volunteers followed for?
- long enough to detect differences in outcome as well as differences in side effects
- often side effects aren’t encompassed in the relative time period
- while longer follow up might be beneficial in terms of detecting side effects, it can also lead to a potentially larger loss to follow up
- this loss-to-follow up is a bias
-
Ascertaining the Outcome
- those evaluating should be blind to treatment assignment
- a PRECISE definition should be used to define the outcome
-
“Intention to Treat” Analysis
- volunteers analyzed in the group to which they were randomized regardless of actual treatment received (eg. if they’re lost to follow up, don’t comply, etc.)
- “best” measure to be used to analyze the results
- the idea is to retain benefits of randomization
-
How can you tell if a new treatment is better than a placebo in a RCT?
compare the two arms using Relative Risk (RR)
-
Relative Risk (RR)
- Outcome Incidence in Experimental Group / Outcome Incidence in Control Group
- for a cohort study, RR can be written as:
- Outcome Incidence in Exposed / Outcome Incidence in Unexposed Group
- a ratio of risks
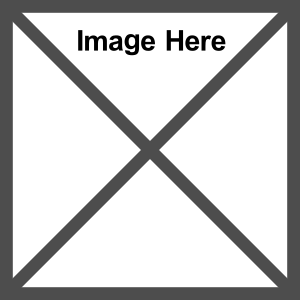
-
RR = 1
- Outcome Incidence in Experimental = Outcome Incidence in Controls
- RR = 1 is also called the “Null Value”
-
RR < 1
- when the outcome incidence is higher in controls than in the experimental group
- the denominator (controls) is bigger, so RR < 1
-
RR > 1
- when the outcome incidence is higher in the experimental group than in controls
- the numerator (experimental group) is bigger, so RR > 1
-
Why are subgroup analysis important?
- they ascertain effect modification
- eg. is the effect of a treatment on an outcome the SAME in all people that participated in the trial (eg. in women & men, young & old, etc.)
- subgroup analyses are important to clinicians because they may identify subgroups in which treatment is helpful or harmful
-
-
What are the 2 overarching types of Epidemiological studies?
- 1. Observational
- 2. Interventional
studies within these categories can either be Descriptive or Analytic
-
How do descriptive case series’ differ from cross-sectional studies?
- in case series there is NO comparison group
- in cross-sectional studies there’s an experimental & control group
-
Descriptive Observational Studies
- Case Report
- Case Series
- Cross-sectional
- Correlational
-
Analytic Observational Studies
- Cohort Studies
- Case-control Studies
-
Descriptive Interventional Studies
- Case Report
- Case Series
- these 2 kinds of studies can ALSO be categorized as interventional because the exposure can be chosen by an investigator
-
Analytic Interventional Studies*
Randomized Control Trial
-
Cohort Studies
- observational studies that are analytic (as opposed to descriptive) in nature
- investigator recruits 2 types of individuals: exposed & unexposed
- the investigator then follows these 2 groups through time & eventually measures the incidence of outcome in each
- eg. twin studies comparing twins who had different exposure levels
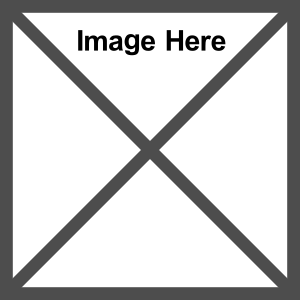
-
Prospective Cohort Study
the investigator starts a study (TODAY) & follows exposed & unexposed volunteers through time (eg. for 10 years FORWARD) & then compares the incidence of outcome in exposed v. unexposed volunteers
-
Retrospective Cohort Study
- the investigator again might start the study today, but then looks BACK in time with the assistance of medical records to determine who’s exposed & who’s unexposed
- the investigator might then again compare current day outcomes in both groups
-
When is a Cohort Study appropriate?
- when you’re interested in incidence rates or predictors MORE than the effects of interventions
- it can be used before a randomized trial is proposed (eg. to generate hypotheses like the effects of hormone replacement therapy or dietary fat)
- when exposure CANNOT be randomized (eg. genes, race, BMI, serum cholesterol, potentially harmful exposures [cigarettes, drugs, pesticides])
-
Is a Cohort Study more or less valid than an RCT?
- a Cohort Study has a LOWER validity than an RCT
- this is mostly because there is no randomization in a Cohort Study
- it’s also more difficult to measure exposure because often we rely on self report
-
What is the goal in regard to Exposure in a Cohort Study?
- to have an ACCURATE measure of true exposure
- definition of Exposure should be clear & concise
- it should be measured with accurate instruments, & the same method/instrument should be used in the exposed & unexposed
- Exposure should be assessed BLIND to outcome (to avoid investigator bias)
-
What is the goal in regard to Outcome in a Cohort Study?
- to have (again) an ACCURATE measure of the true outcome
- a clear & precise definition is needed
- outcome should be measured with an accurate instrument
- potential sources of outcome data include disease registries, medical records, death certificates
-
What are some advantages of Cohort Studies?
- they work well for exposures that can’t be randomized (genes, drug use)
- they’re good for RARE exposures
- they can assess multiple outcomes
- they can generate Incidence data
-
What are some advantages of Retrospective Cohort Studies in particular?
- they’re good for studying diseases with long latency
- they take less time to complete
- are less expensive
- (less time & resource intensive)
-
What are some DISadvantages of Cohort Studies?
- the exposure can’t be randomized
- they’re bad for rare OUTCOMES (because you have to follow many many people over a long time to see the outcome)
- - this long follow-up time to observe outcomes can also lead to loss to follow-up & a change in someone’s exposure status
- they can be EXPENSIVE because of the number of years needed to follow the cohorts
- subjects need to be free of the outcome at the start of the study & sometimes that can be hard if diagnosis can’t easily be done or isn’t clear-cut
- in a retrospective cohort study, the data may not be available or adequate
-
Relative Risk (Risk Ratio, Rate Ratio) in a Cohort Study
- can be used as a measure of association between exposure & outcome
- the probability (risk) of developing the disease if exposed compared to the probability of the developing disease if unexposed
- RR = Incidence of Disease if Exposed / Incidence of Disease if Unexposed
- unexposed (controls) are in the denominator
-
Cohort RR > 1
- exposure promotes the outcome
- eg. a larger # of people got the disease (outcome) if they were exposed
- larger numerator
-
Cohort RR < 1
- exposure prevents outcome
- eg. a larger # of people DIDN’T the disease (outcome) if they weren’t exposed
- larger denominator
-
RR = 1
- the exposure had no effect of on the outcome
- the same proportion of people got the disease (outcome) in both the exposed & unexposed groups
- RR = 1 is the null value
-
Attributable Risk (Excess Risk/Risk Difference)
- Incidence in Exposed - Incidence in Unexposed
- it’s the incidence DUE to exposure, because it’s the difference between the two incidences
- it compares the incidence of disease (outcome) in the exposed group & the incidence of outcome in the unexposed group
- is another measure of association between exposure & outcome of interest
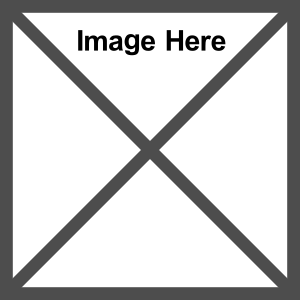
-
Number Needed to Treat
1 / Attributable or Excess Risk
this is the # needed to treat to prevent 1 occurence of a disease (eg. stroke)
-
Relative Risk v. Attributable Risk (RR v. AR)
- RR can be useful in helping an individual understand their personal risk of disease by continuing to expose themselves to an exposure
- RR might be what’s shared with a patient
- AR is important in public health advocacy work (eg. when talking with legislators or policy makers)
- AR helps you understand the # of cases that can be averted, or can be converted into the cost of a disease in a population
-
Bias
- the distortion of a study’s results
- the results of the study don’t reflect the truth when there’s systematic error in the measurement of an association between 2 variables
-
What are different types of Bias?
- Confounding
- Selection Bias
- Information Bias (random + non-random)
- Loss to Follow-up
- *unlike with confounding, the other sources of bias CAN’T be corrected during analysis & MUST be avoided in the study design
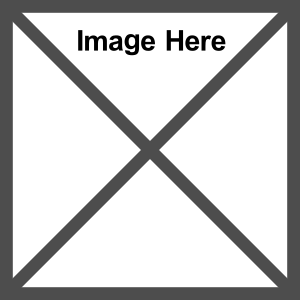
-
Random (Non-differential) Misclassification of the Exposure
any misclassification of the exposure that’s the SAME in both outcome groups
-
What are some examples of Random Misclassification of Exposure?
- 1. ALL volunteers lie about substance abuse → underestimate of exposure
- 2. ALL volunteers over-estimate daily physical activity → overestimate of exposure
- 3. ALL subjects have trouble recalling average red meat consumption
- with these 3 examples, the bias is ALWAYS toward the NULL
- there’s a “watering down” of the association
-
Random (Non-differential) Misclassification of the Outcome
any misclassification of the outcome that’s the SAME in both exposure groups
-
What are some examples of Random Misclassification of Outcome?
- 1. an investigator may UNDER-diagnose outcomes in ALL volunteers (fewer people will have the outcome of interest)
- 2. 1. an investigator may OVER-diagnose outcomes in ALL volunteers (more people will have the outcome of interest than should)
- 3. a disease is difficult to conclusively diagnose (eg. MI)
- again, the bias is ALWAYS toward the NULL (there’s a watering-down effect)
-
Non-random (Differential) Misclassification of the Exposure
any misclassification of the exposure that’s DIFFERENT in outcome groups
-
What are some examples of Non-random Misclassification of Exposure?
- 1. mother’s with babies born with FAS LIE about alcohol use
- 2. volunteers who haven’t had an MI over-estimate physical activity
- 3. investigators over-estimate exposure in people with a disease & under-estimate exposure in people without a disease
- bias can either be toward OR away from the NULL
-
Non-random (Differential) Misclassification of the Outcome
any misclassification of the outcome that’s DIFFERENT in exposure groups
-
What are some examples of Non-random Misclassification of Outcome?
- 1. investigators under-diagnosing an outcome in people who’ve had surgery v. people who haven’t had surgery
- 2. investigators over-diagnose a disease (outcome) in those who were exposed v. people who weren’t exposed
- 3. investigators UNDER-diagnose people in the exposed group & OVER-diagnose people in the unexposed group
- can lead to bias toward or away from the null
-
How can non-random misclassification of exposure or outcome be avoided?
by BLINDING investigators to both the exposure & outcome of interest
-
-
External Validity (Generalizability)
the ability to apply the findings from our study population to a larger population
-
Internal Validity
- the degree to which a study’s findings represent a true reflection of the exposure-outcome association in the population
- how close the estimated relative risk in a study is to the true (but unknown) relative risk
- internal validity = absence of bias (bias = “distance from the truth”)
-
What are the 3 steps of assessing internal validity of a study?
- 1. Rule out confounding
- 2. Rule out other sources of bias
- 3. Rule out chance with statistical tests
-
RCTs & Internal Validity
double-blind RCTs are considered the “gold standard” of epidemiologic study designs because the internal validity (absence of bias) is greater than in other study designs
-
What 3 things must a variable be to be considered a confounder?
1. it must be a risk factor for / associated with the outcome
2. it must be associated with the exposure, or unbalanced in the exposure group
3. it must NOT be on the intermediate path between the exposure & outcome, aka it can’t be a mediator on the causal pathway between the exposure & outcome
-
Framingham Heart Study & Menopause
researchers showed that menopause increased a woman’s risk for heart disease
what was actually happening was that age was acting as a confounder, creating the ILLUSION of a positive association between menopause & CHD
the criteria: age IS a risk factor for CHD, age is UNBALANCED across exposure groups (pre/postmenopausal women tend to be different ages), & age is NOT on the causal pathway (like endogenous estrogen, which would be)
-
Risk Factor
- an attribute or exposure associated with an increased or decreased probability of a health-related outcome
- not necessarily a direct cause of disease
- eg. vaccines are an example of a “risk factor” that may prevent disease.
- also called exposure, predictor, or determinant
-
How do you address confounding in relation to study design?
- you can Randomize individuals into the 2 arms of the study
- you can Restrict which subjects you include (eg. if sex is a cofounder, just do the study in men)
- you can MATCH subjects on specific characteristics that you KNOW to be confounders (eg. sex, age, race, ethnicity)
-
How do you address confounding in relation to study analysis?
via Stratified analysis & Multivariable analysis
-
Matching
- a way to avoid confounding in the study DESIGN
- eg. TWIN studies
- if sex, ethnicity, & age were confounders, then matching eliminates their ability to confound
-
Effect Modification (Interaction)
a factor OTHER than the exposure of interest (or even the disease) that can modify the exposure-disease association
-
Confounding v. Effect Modification
Conf: look at a difference between crude & adjusted RR/OR (eg. within the same variable but different when adjusted for various other variables)
EM: look across subgroups (strata); applies to variable of interest (eg. age)
-
-
Scattergram
- visually summarizes the relationship between two continuous variables
- eg. vitamin D serum levels v. vitamin D supplementation
-
RR, OR, & Pearson R
- RR & OR are single numbers used to quantify the relationship between 2 binary variables
- when you have 2 continuous variables, as in this example, the relationship can also be quantified by a single number: r
-
r (Pearson Product-Moment Correlation Coefficient)
- quantifies the magnitude & direction of LINEAR relationships between 2 CONTINUOUS variables
- is the average of the product of the Z-scores between the two variables of interest
- has NO units
- range = - 1 → +1
-
Pearson Correlation Coefficient (r) Values
- 0: no linear relationship
- +1: a perfect positive linear relationship (positive means as the values of 1 variable increase, so do the values of the other variable)
- -1: a perfect inverse linear relationship (negative means as the values of 1 variable increase, the values of the other variable decrease)
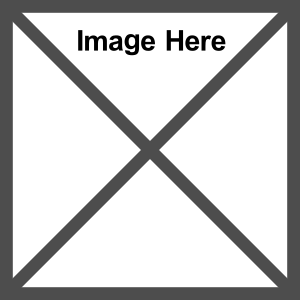
-
Regression Analysis
a statistical tool for evaluating the relationship of one or MORE independent variable (predictors) to a SINGLE continuous dependent (outcome) variable
in addition to evaluating relationships, regression analysis can also be used to predict outcomes (via deriving prediction equations)
-
Simple Linear Regression
- used to fit a straight line (describe & predict a linear relationship) through points on a Scattergram
- done in relation to 2 variables (X & Y axis)
- the straight line is derived mathematically so the same # of data points lie above & below the line
-
Simple Linear Regression Line of Best Fit
- Y = βo + β1X
- Y: the predicted value (outcome)
- Bo: the INTERCEPT/cOrrelation coefficient
- B1: the s1ope coefficient
-
Vitamin D Eg. Line of Best Fit
for every 1 unit of supplemental vitamin D taken, the estimated increase in vitamin D serum levels is 0.0249 units
Y = 64.7 + 0.0249X
-
What are the 2 distinctions between slope coefficient & relationship coefficients (worth remembering)?
1. the s1ope coefficient β1 has UNITS, the cOrrelation coefficient doesn’t
2. the cOrrelation coefficient βo just assesses the strength of the relationship; it doesn’t describe how the dependent variable changes in relation to changes in the independent variable
-
What kind of relationship is there between slope & correlation coefficients?
a 1 to 1 relationship
-
Different Values of β1 (S1ope Coefficient)
-
R2 (Coefficient of Determination)
- proportion (percentage) of variation in the outcome variable that can be explained by the exposure
- ranges from 0 → 1 (0 → 100%)
eg. if R 2 = 0.73, then 73% of the variability in the outcome can be explained by the exposure
-
Multiple Linear Regression
used to describe & predict a linear relationship between 1 dependent variable & 2 or more independent variables
the adjusted r2 corresponds to one from a multiple linear regression, & sometimes explains more variability (the value increases)
-
Multiple Linear Regression Line of Best Fit
Y = βo + β1X1 + β2X2 + β3X3…
-
What are the benefits of a Multiple Linear Regression?
it can assess multiple exposures
it can assess potential confounding
-
β Estimates in Multiple Linear Regression
- the β values correspond to each variable - so for every 1 unit that the independent variable changes by, the dependent variable (what you’re measuring) changes by the β coefficient that corresponds to that specific independent variable
- this β coefficient exists after adjusting for all variables in the regression model
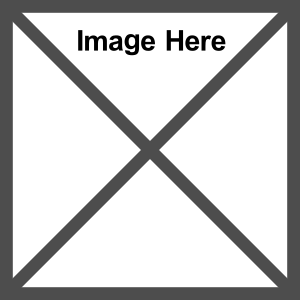 - vitamin D eg.: for every 1 ounce of fish eaten, vitamin D serum levels increase by 2.01 (the individual β coefficient for the variable “fish intake”)
-
When is a Logistic Regression used?
- when the outcome is binary (yes/no, present/absent - is an extension of the 2 by 2 table)
- can be used to calculate adjusted odds ratios & relative risks for more than 1 extraneous variable
- to generate prediction equations in the case of binary outcomes
-
Log Regression Equation
- π(x) = eβ1+β1x / 1+ eβ1+β1x
- βo & β1: unknown parameters
- x: exposure
- π: proportion of the outcome (1=yes, 0=no)
-
How is the logistic model written?
as a non-linear equation to ensure the outcome is bounded between 0 & 1
if logistic regression is used to predict the risk of outcome, the risk estimates can’t be less than 0%
-
How do you calculate the β coefficients for a logistic regression?
- convert the log equation to a linear one via a logit transform
- ln[odds] = ln [π(x) / 1 - π(x)] = βo + β1
-
When is a Time to Event Analysis used?
when the time to an event is AS important as whether the event occurs or not
-
Time to Event Analysis
- accommodates varying lengths of follow-up
- outcome of interest is the TIME until an event occurs rather than whether it does or doesn’t occur
-
Why might there be varying lengths of subject follow up?
- 1. staggered entry into the study
- 2. subjects might drop out of the study, are lost to follow up, or may die
- 3. subjects who don’t have an outcome by the end of the study
-
Physician Waiting Room Example
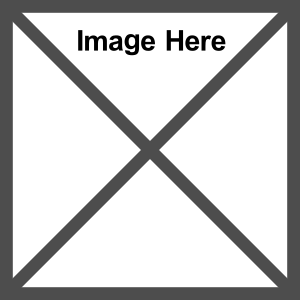 - F: saw physician (outcome achieved)
- L: lost to follow up at noted time
- C: censored (outcome not achieved in specified time period)
- question to ask: What is the rate (risk, chance, likelihood) of being seen by a physician (outcome) within __ minutes of arriving at the doctor’s office?
-
Incidence Density
- # of new cases in a specified time period / total number of units of person-time
- a simple but MORE precise measure of incidence than cumulative incidence
- the advantage of ID is that it accounts for unequal follow up & loss to follow up
-
Incidence Density with Doctor’s Office
- 12 total patients
- 3 patients were seen within 15 minutes of arrival
- minutes waited by everyone = 146
- ID = 3 / 146 = 0.0205
- 0.0205 * 100 = 2.05 patients per 100 patient-minutes
- 2.05*15 = 30.8 patients will be seen per 100 patient-minutes within 15 minutes
-
Doctor’s Office Incidence Density Interpretation
there’s a 30.8% CHANCE a patient will be seen by a physician within 15 minutes of their arrival
-
Kaplan-Meier Event-Free Survival Curve
- an approach to display the cumulative proportion of participants who did NOT experience an outcome event over time
- each step plotted on a K-M curve represents an outcome event
- because K-M analysis & corresponding curve accounts for varying lengths of follow up, the K-M estimates are VERY close to Incidence Density
-
Logrank Test
- used to compare Kaplan-Meier curves between 2 or more groups
- the comparrison is made across the entire event free survival curve & not at any particular period of time
-
Cox Proportional Hazards Regression
does the same thing that a logistic regression does (accommodating multiple exposure variables [including potential confounders & effect modifiers] for time to event data
-
Hazard Function
- h(t) = h0(t)eβ1x1 + β2x2…
- h(t): hazard @ time t
-
Logistic Regression is an extension of the __ _____ ____, & Cox Regression is an extension of the _________________
- Logistic Regression is an extension of the chi-square test
- Cox Regression is an extension of the event free survival analysis
- while the log regression model yields odds ratios, a Cox regression model yields hazard ratios
-
How can hazard ratios be interpreted?
- as Relative Risks
- hazard ratios are byproducts of the Cox regression model
-
-
Case-control Study
- a type of observational analytic study in which subjects are selected based on their disease status
- subjects are classified as Cases (having the disease) or Controls (not having the disease)
- cases are identified by the outcome/disease being clearly & precisely defined
- Good for rare OUTCOMES (diseases); Bad for rare exposures
-
How should case & control status be assessed?
- investigators should be blind to a person’s exposure status when assessing if the person is a case
- similarly, exposure should be assessed blind to outcome
-
Why are incident (new) cases better than prevalent cases?
prevalent (old + new) cases may be survivors & therefore may not be representative of “typical” cases
-
What cannot/is not calculated in a Case-control Study?
- INCIDENCE, because we started with cases (people who already have the disease of interest)
- therefore relative risk can’t be calculated either
-
So, what is the only measure of association possible in a case-control study?
Odds Ratio
OR is a good estimate of the relative risk when the disease of interest is rare (<10%)
-
Remember: Cohort Study
- in both a prospective & retrospective cohort study, disease incidence can be calculated → RR can be calculated (as can OR)
- Case-control can ONLY use OR
-
Odds Ratio (OR)
odds that a case was exposed / odds that control was exposed
-
What are the advantages of Case-Control Studies?
- Good for rare diseases
- Allow for evaluation of multiple exposures
- Efficient (re: time & cost)
- Avoid potential ethical issues of an RCT (eg. can’t randomize subjects to a harmful exposure like smoking)
-
What are the disadvantages of Case-Control Studies?
- they’re BAD for rare exposures
- Comparability of cases and controls might not be given
- Can’t generate incidence data because time isn’t known
- there’s potential Selection bias, Interviewer bias & Recall bias (are all controls really free of the disease?)
-
Selection Bias
- caused by how the study subjects were selected for the study
- results in the association between the exposure & outcome not being representative of the target population’s true association
- to avoid selection bias, the exposure & outcome shouldn’t both be mentioned in recruitment material
-
What type of study cannot have Selection Bias?
- RCT
- because subjects join the study before they know their exposure status & because the outcome has not yet occurred
-
Recall Bias
- Cases (who have experienced an adverse health outcome) may be more likely to recall exposure histories than controls
- eg. cancer cases recall pesticide exposure more readily than people without cancer
- it can attenuate association between disease & exposure towards the null or exaggerate association away from the null
- a differential (non-random) misclassification of the EXPOSURE
-
In what kinds of studies is Recall Bias found?
- Retrospective Studies only
- because outcome has already occurred when the Exposure is assessed
-
Advantages of Case-control v. Cohort Studies
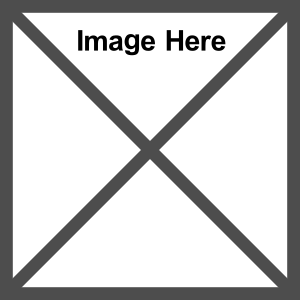 - Case-control: recruit people based on their disease (outcome) for the outcome arm then find controls to match that don’t have the disease
- Cohort: observational study, recruit people just based on whether or not they have or don’t have a certain exposure → outcome is assessed “later”
-
Disadvantages of Case-control v. Cohort Studies
-
Why are all observational studies subject to confounding?
because there’s NO randomization
[small RCTs are more subject to confounding than large RCTs]
|
|